Beyond privacy regulations: an ethical approach to data usage in transportation
&w=256&q=90)

Privacy regulations have completely changed the way companies approach data. But adapting to regulations is not enough. Privacy-by-design solutions such as federated machine learning go beyond current regulations and into the world of ethical data-usage.
The world now revolves around connectivity. From the smartphone sitting your pocket, the TV in your living room and now the car that you drive, it is almost impossible to live without connected technology. In fact, the International Data Corporation (IDC) estimates there will be 41.6 billion of such devices, generating 79.4 zettabytes globally by 2025.
The application of this vast amount of data is almost limitless and represents a gold mine of potential. Using data, companies can deliver extremely personalized services that take into account the exact needs of the driver.
But as the ecosystem is growing, so is the concern over data privacy and security. Users now seek out personalized products they can trust that does not compromise their personal information. New privacy regulations such as the General Data Protection Regulation (GDPR) in the EU and the California Consumer Privacy Act (CCPA) in the United States have forced companies to rethink how they approach data and adapt their processes accordingly.
But it is not enough to react to regulations after they have been introduced. Instead, the industry must explore solutions that always respect the user’s privacy while looking to accommodate an ever-changing legal landscape.
The connected car data revolution
Connected car technology has transformed the transportation industry. Modern cars are equipped with complex electrical systems that collect data from a multitude of sensors and use it to improve the driving experience. Car manufactures can analyze user preferences and behavior to improve and offer extremely personalized services from infotainment devices, location-based services to wireless vehicle diagnostics.
Location-based navigation and route guidance not only make drivers’ lives easier through considering daily habits, weather and traffic conditions, but can also be used to plan the most ecological route on any given day.
In the transportation sector, the most sensitive private information is the user’s location. This information can reveal highly personal information about a person’s daily habits, workplace and home address. Companies must guarantee that personal data is handled according to the latest privacy regulations. But more than simply abiding, it is important for privacy to be at the core of how these products and services are designed.
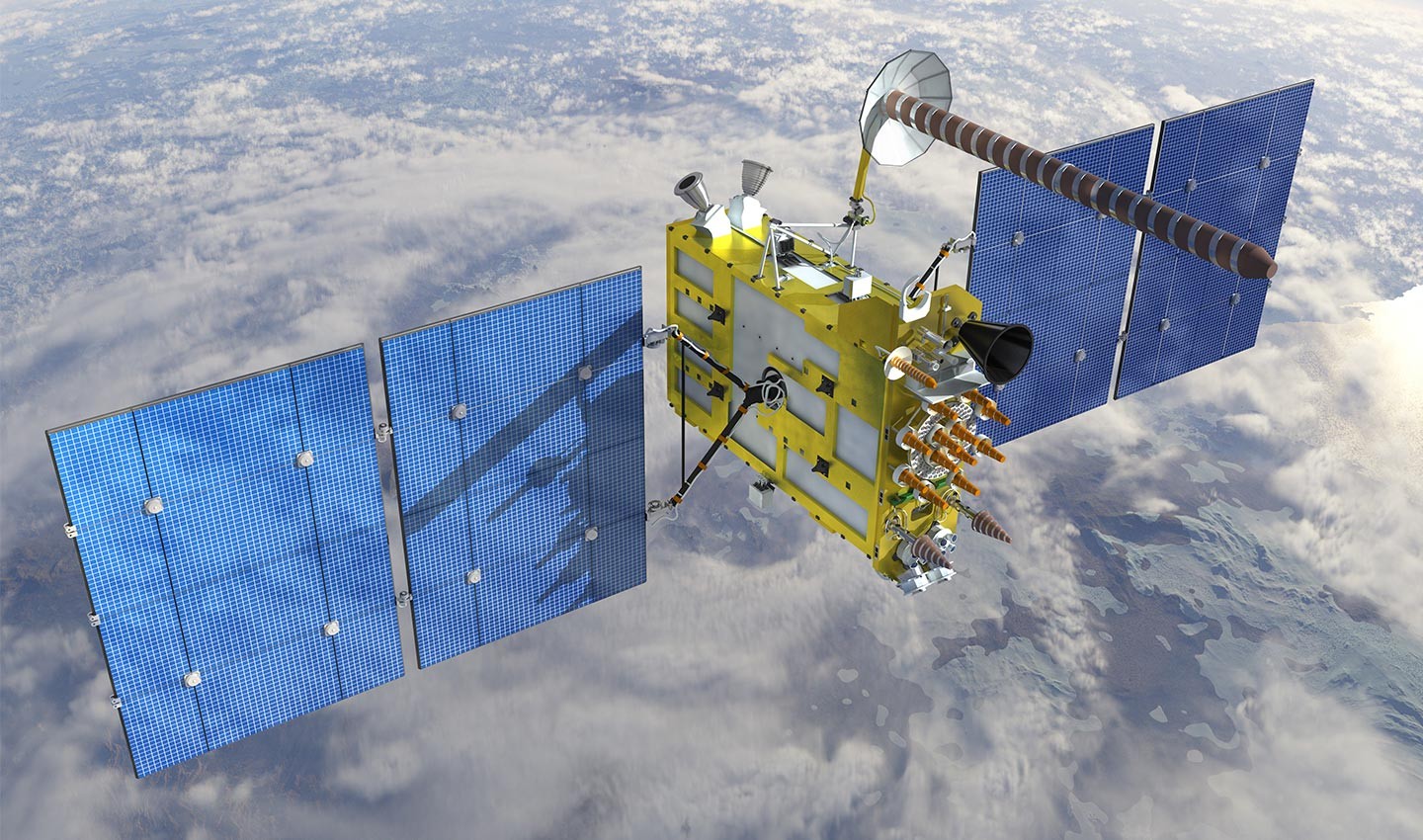
For TomTom and other industry companies who want to stay ahead of the regulation curve, applying privacy-enhancing technologies (PET), is the next step towards a more secure and ethical usage of connected car data.
What are privacy-enhancing technologies?
Privacy-enhancing technologies are methods that allow for processing privacy-sensitive data securely with the goal of protecting the user’s personal information. They are an element of the privacy-by-design paradigm that intends to create systems and processes that at their core mitigate privacy risks without compromising the quality of the data-driven decisions.
Federated machine learning
One of the most promising PET solutions for the automotive industry is federated machine learning.
In traditional data analysis solutions, all user data is moved to a centralized remote server where the information can be analyzed by machine learning algorithms. But the rise of privacy regulations means companies now consider how to anonymize privacy-sensitive data and execute deletion at the customer's request. Without this data, it becomes difficult to perform advanced analytics with classical methods.
Federated machine learning is considered a privacy-aware machine learning method, where devices that collect data, perform computations locally and send only the aggregated results to a central entity. This adds a layer of protection to a user’s personal information while still allowing carmakers access to crucial information that can be used to improve products and services.
From a company perspective, federated learning ensures they can deliver the best tailored services, reduce cloud infrastructure costs and stay ahead in an ever-changing legal landscape.
“Johannes M. van Hulst et al.
How is TomTom researching federated machine learning?
Although classic machine learning solutions are currently used, we have identified three key use cases that would benefit from a federated learning approach.
Destination prediction is the process of automatically predicting the destination of the driver without requiring manual tasks. Traditionally the users trip history is moved to a backend server from which machine learning algorithms infer patterns to give the user potential destination options. In the federated setting, privacy-sensitive data can be stored locally on the user’s device. This mitigates privacy risks but does not compromise on the traditional benefits of machine learning, such as the ability to infer knowledge of one user’s patterns to improve the experience of another.
Route prediction aims to provide route information while taking into consideration the past trips and preferences of the driver. Through performing route prediction computations locally, the device can use the personal preferences of the customer. TomTom can use local data to predict the user’s preferred route from to work in the morning or understand that the driver doesn’t mind adding a few minutes to their journey if it means they will avoid traffic jams.
Another aspect that could benefit from federated machine learning is the estimated time of arrival (ETA). To calculate the ETA, TomTom uses multiple variables including traffic, speed limits, road closures and weather conditions. With personal data kept locally, federated machine learning has the potential to both protect the user’s information and take into consideration the personal driving style of every driver, improving ETA accuracy on a personal level.
Towards an ethical approach to privacy data
TomTom is always striving towards the most secure privacy designs to protect our user’s data with strict compliance to regulations. Federated machine learning offers an opportunity to create a new way of incorporating privacy-by-design approaches into products.
As connected devices transmit more and more data every day, TomTom helps customers get the most out of their data while always respecting their sensitive private information.
Want to learn more?
Download our paper, “Beyond privacy regulations: an ethical approach to data usage in transportation”, to find out more about federated machine learning.
People also read
)
Data privacy in the era of the connected car
)
How we make our HD maps
)
How to structure machine learning work effectively
* Required field. By submitting your contact details to TomTom, you agree that we can contact you about marketing offers, newsletters, or to invite you to webinars and events. We could further personalize the content that you receive via cookies. You can unsubscribe at any time by the link included in our emails. Review our privacy policy.