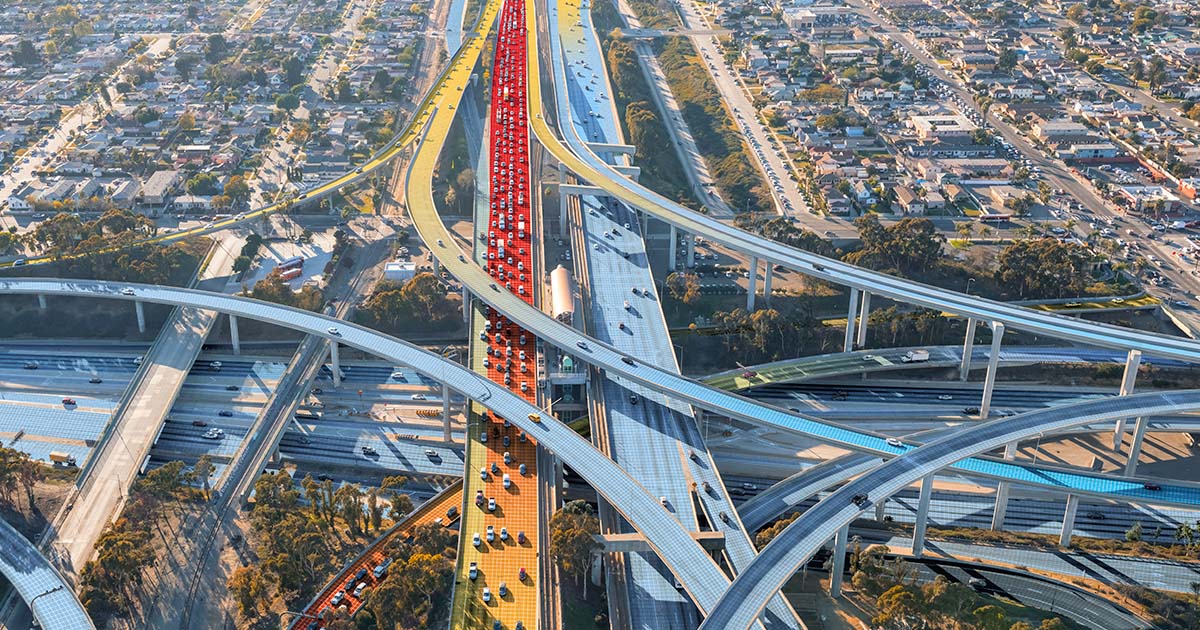
Knowing about traffic flow and density is important to on-demand platforms, but it’s just one ingredient of a full mapping suite. Successful applications in this space take advantage of traffic data alongside many other mapping features to ensure the best possible experience for users.
When we think about traffic, it’s easy to focus on accidents, congestion, and patterns of travel. All of these have a big impact on how long it takes drivers to get from point A to B, but these changes also affect what’s happening in an on-demand app.
An application should be able to adapt to changes in traffic to support route planning. However, this then affects the driver’s navigation experience and what the customer sees on their device.
While these components are individual parts of the journey, they work together to reduce the time all parties spend in congestion and to create a user experience that provides total clarity as to when the customer or their delivery is going to arrive.
Planning the perfect traffic-informed route
Route planning is one of the best ways to obtain an accurate estimated time of arrival, aka, an ETA. Ideally, this ETA is the same for both user and driver throughout the delivery process.
While a user interacts with an application, route planning might occur in a couple of different places: when a customer requests a rideshare pickup, and when a driver is matched with a passenger or delivery (or series of deliveries or drop-offs). It will then repeat for the driver when they are matched with subsequent passengers while on their current trip.
The optimally laid-out route takes into account existing road conditions, known traffic incidents, and historical and real-time traffic and road information. That’s a lot of detail, so let’s break down what’s under the hood of an example app whenever a user requests a ride or delivery, built with TomTom Maps APIs:
1. Evaluating road hazards at the time of the request
On a map, the Traffic Incidents API overlays visual indications of accidents, road or lane closures, and other dangers on the road, such as ice. This portion of the Traffic API can return the magnitude of the event, the time it happened, and provide visual feedback that is easy to understand for all users (both drivers and passengers). These road hazard details may be something the user sees when first looking at the map portion of the application on their phone, so they can be aware of their surroundings before summoning a ride.
2. Taking stock of traffic
When a request is made, a quick evaluation of traffic occurs to determine the most congested areas. This is known as the Traffic Flow API and uses familiar-colored overlays of lanes to indicate how slow or fast traffic is moving on a particular stretch of road. Before using this information to help plot the fastest route, the map might display traffic incidents and flow within a radius of each point, origin, and destination.
3. Planning routes using Speed Profiles
In this key step, the Routing API uses TomTom’s routing engine to build a journey using not only traffic information from above, but also other data such as that of Speed Profiles. Where TomTom Traffic reports current speeds and conditions, updated every minute, Speed Profiles utilizes historical traffic data from the past year to note the actual average speed for a given stretch of road, at each time of day. This is key when comparing speed limits to actual speed traveled on certain roads – some might be habitually congested, and some might have higher regular travel speeds than expected.
These components represent a considered formula perfected over years of analyzing key information related to how and when traffic moves. It all happens behind the scenes in just a few seconds when a driver and customer are matched for a trip.
When all these components come together, they produce a route which is most likely to adhere to its original ETA – both for the driver arriving for pickup (of person or food delivery) and for a passenger reaching their destination.
In the unpredictable world of rush hour traffic, last-minute appointments, and dinner dates, selecting the path of travel least likely to create unforeseen delays goes a long way to keeping drivers, passengers, and delivery recipients happy and coming back to the app.
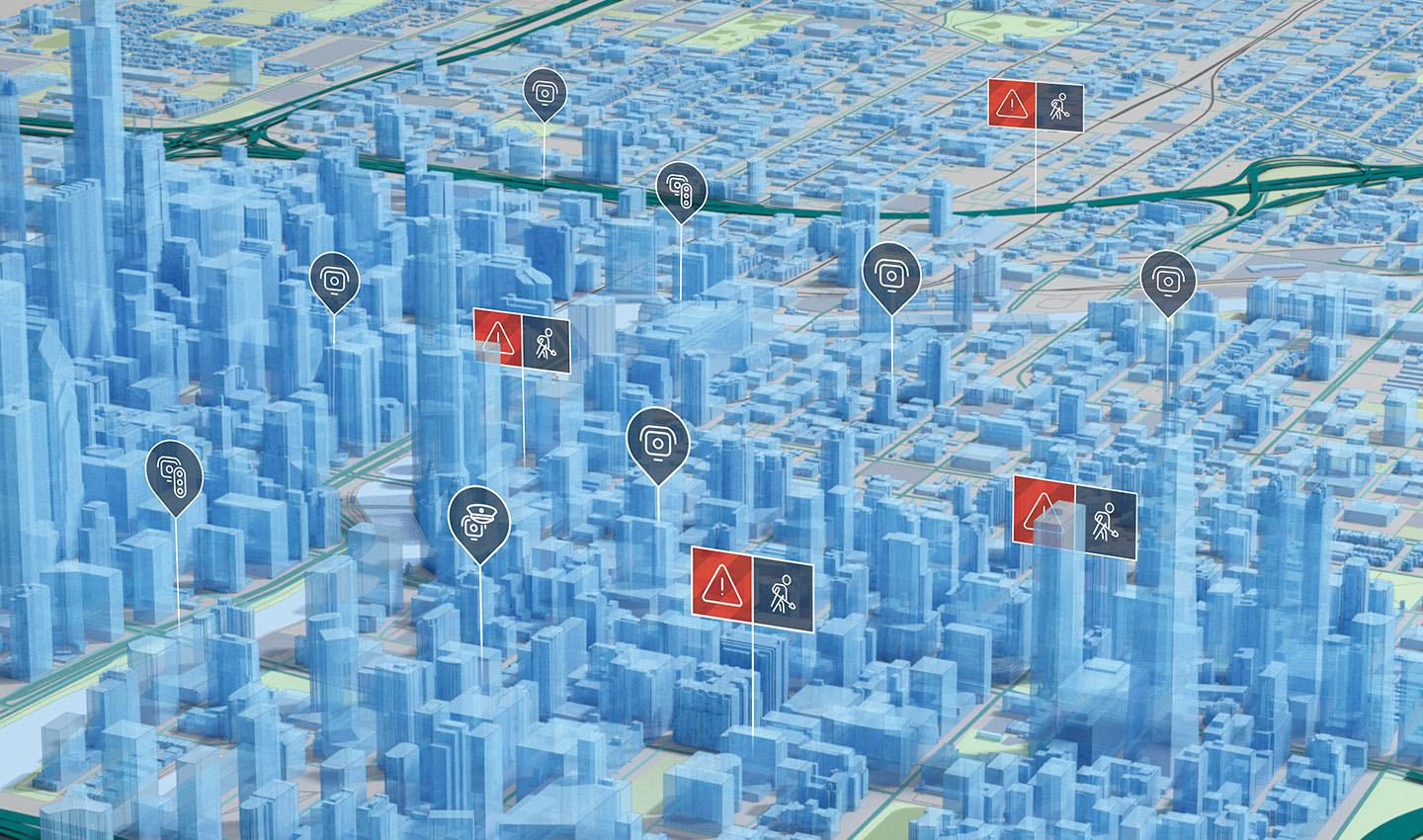
Traffic incident details support users by helping them make informed travel decisions.
The relationship of traffic and city centers for rideshare drivers
This experience for customers – those doing the ordering, riding, or summoning – is perhaps most prized, but shouldn’t come at the expense of the drivers’ experience. It’s important to recognize that drivers and riders might not share the same exact mapping experience at the same time, and that’s for good reason.
Within rideshare models, drivers typically collect serial orders, which means an application may be constantly searching for nearby drivers, whether or not they are already on a trip. You’ve probably seen this in motion if you’ve driven for or taken many ride-shares; in the last few minutes or miles before drop-off, a driver is often pinged to take on a subsequent trip. The goal is to minimize their time and fuel use by picking up rides or orders near their drop-off location.
This is why it can be even harder to plot long-distance fares, and instead it’s logical for mobility applications to focus on city centers. Trips to less populated areas mean that the driver is less likely to have a ride request come in after their drop-off; without a passenger, the driver is then going without fare while making their way back to a city center with more users.
When drivers collect subsequent rides in city centers, they also have the luxury of operating within nearby access to many more major roads and freeways, allowing the routing of many possible detours from trouble spots. These major roads usually have more regular traffic patterns, bolstering the accuracy and reliability of traffic predictions. With the addition of more physical room for accidents to be cleared and allow cars to move around incidents, they allow for fewer holdups and represent a denser field of data as they are more widely traveled.
Creating an optimal experience around time estimation and traffic for rideshare drivers is key, but it doesn’t complete the ideal user experience yet. Next, let’s focus on the components that help this application retain users from short-range delivery applications.
How traffic data supports short-range delivery
In the example above, we talked about what happens under the hood after a passenger makes a request which is sent to a driver. When you open a delivery app, typically there are estimates already waiting for you, giving you immediate insight into how far away your delivery might be before you take any action.
When traffic predictions fail drivers, they also fail customers – however, when they support drivers, they create seamless transitions that go unseen by users. Better data leads to better informed estimates and enables smarter use of trip time for drivers. A popular example of this is making multiple deliveries along the same route for short-range food delivery.
From a delivery driver’s perspective, delivering multiple food orders along a similar path of travel saves fuel, and allows them to take new orders faster to maximize their working time, just like our rideshare driver. Optimizing deliveries along fastest routes is especially important in the case of multiple orders at once, so that orders delivered farthest away from the driver still meet customers’ expectations.
By comparison to the driver’s experience flow, the user’s might show lags while a driver drops off a nearby food order between the restaurant and their final delivery spot. This could be the result of avoidable traffic conditions. If traffic along routes is estimated with multiple stops in mind, the driver will be able to adapt their journey to create the best possible delivery time for all of their customers in that trip.
Ideally, adjustments to the driver’s delivery route made in the interest of traffic means that no customer will notice delays with their order. Similarly, drivers can communicate with each customer and provide real-time updates if necessary, working to preserve the user experience to keep customers coming back to the app.
Traffic-based decisions drive better results
Mobility applications require building traffic-optimized experiences both for drivers and customers, as each trip represents an exercise in both parties evaluating how well ETA calculations stand up to their promise.
Building these positive experiences early on, backed by TomTom’s traffic data and Maps APIs, can reinforce that trust you need to build up your user base and take your mobility ideas to the next level.
Ready to build a better in-app experience with TomTom Maps APIs? Visit our product page for more details.
People also read
)
3 ways to excel at predicting traffic incidents
)
The ETA conundrum
)
Crowdsourcing, fleets, and the last mile: solutions for small businesses
* Required field. By submitting your contact details to TomTom, you agree that we can contact you about marketing offers, newsletters, or to invite you to webinars and events. We could further personalize the content that you receive via cookies. You can unsubscribe at any time by the link included in our emails. Review our privacy policy. You can also browse our newsletter archive here.